Federated Learning: The Future of AI for Enterprise Leaders
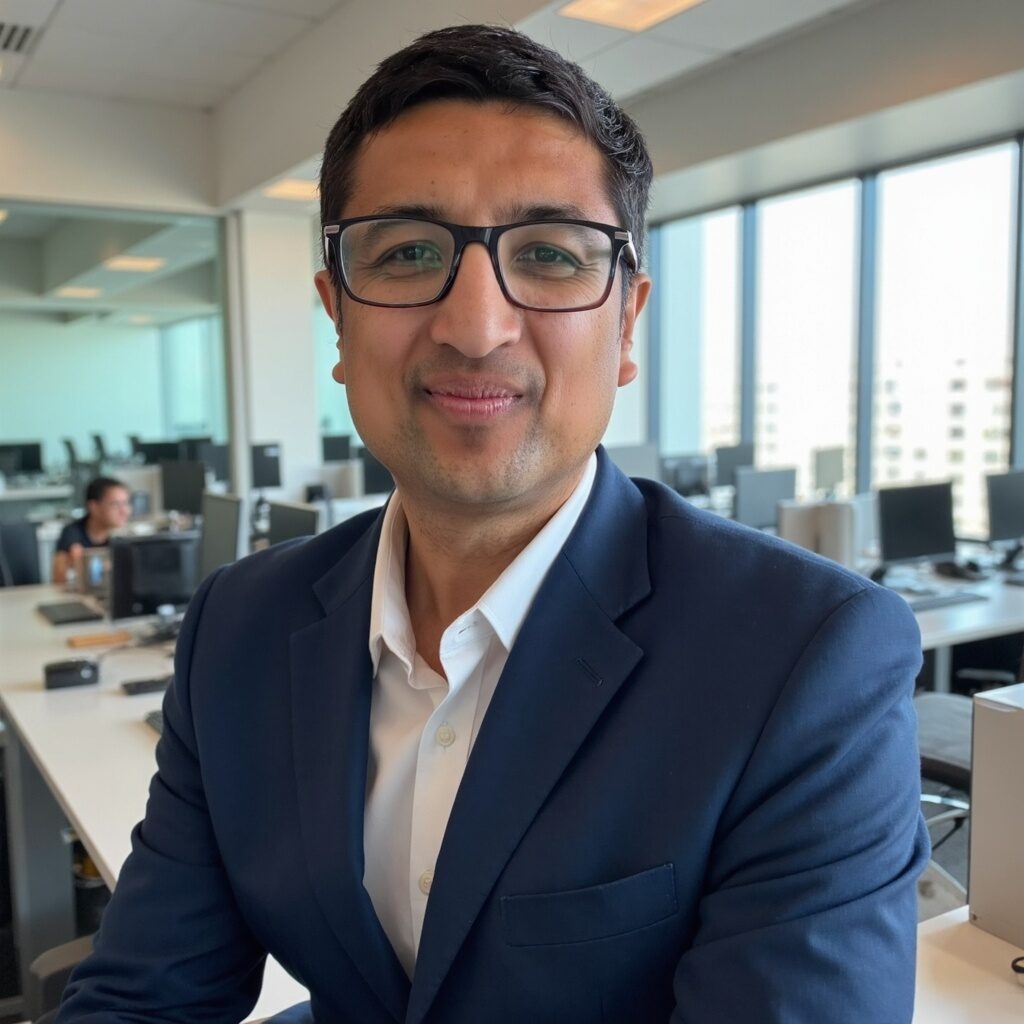
By: Ashish “Monty” Mohanty, SVP at Jade Global
Federated Learning: Impacting AI and data-driven decision making
In the age of data-driven decision-making, enterprises are grappling with a paradox: how to unlock the potential of vast, distributed datasets while preserving privacy, maintaining compliance, and ensuring security. Traditional approaches to AI development rely on centralizing data, but this model is increasingly unsustainable in a world governed by strict privacy laws like GDPR, HIPAA, and CCPA. This is where Federated Learning (FL) can create immense value for organizations. FL is an impactful AI model that can enable organizations to harness distributed data without ever moving it.
For executives (CEOs, CIOs, and CTOs), federated learning is not just a technical advancement; it’s a strategic imperative. It allows enterprises to develop smarter, more collaborative AI systems while safeguarding sensitive data and meeting global regulatory requirements.
Redefining AI in Privacy-First World
Federated learning flips the traditional AI model on its head. Instead of gathering data from various sources into a centralized repository, FL trains models directly where the data resides. These decentralized systems send only encrypted updates—such as model weights or gradients—back to a central server for aggregation. The targeted raw data never leaves its source, ensuring security and privacy.
This approach resolves a critical challenge for enterprises: how to derive value from data stored across diverse systems, geographies, and entities without violating privacy laws or exposing sensitive information. For CEOs, this means scaling AI capabilities while maintaining customer trust. For CIOs and CTOs, it offers a secure, scalable framework for deploying advanced AI systems in compliance with evolving regulations.
Transforming Industries with Federated Learning
Federated learning is already reshaping industries, unlocking new possibilities in sectors where privacy and security concerns have traditionally stifled innovation.
In finance, global banks face the dual challenge of combating fraud and complying with strict data protection laws. Fraud detection relies on recognizing patterns across millions of transactions, often spanning multiple countries. Federated learning enables banks to collaboratively train models on local data without sharing sensitive customer information. For example, a large regional bank based out of the West Coast implemented FL to detect fraudulent activity by aggregating encrypted updates from local branches. The result was a globally informed model that improved fraud detection accuracy while adhering to regional data regulations like GDPR.
In healthcare, the need for collaboration is critical. Hospitals, research institutions, and pharmaceutical companies generate vast amounts of patient data, but privacy laws such as HIPAA limit their ability to share it. Federated learning has emerged as a game-changer. A network of hospitals in Texas used FL to develop a model for early cancer detection. By training locally on imaging data and sharing only anonymized updates, these hospitals created a more accurate diagnostic tool that respected patient privacy and complied with stringent regulations.
In retail, personalization drives customer engagement, but consumers are increasingly wary of how their data is used. Federated learning allows retailers to create recommendation engines that analyze customer behavior locally—on their devices—without centralizing sensitive data. A global retailer recently adopted FL to train a model that provided personalized shopping recommendations. By keeping the data on customers’ devices and sharing only encrypted model updates, the retailer enhanced personalization while earning customer trust and complying with privacy regulations like CCPA.
Even in oil and gas, where operational efficiency is paramount, FL is delivering value. This sector relies on data from geographically dispersed assets, such as rigs and refineries, to optimize operations. Centralizing this data for AI training is often impractical and risky. One large oil and gas company headquartered in Texas used FL to develop a predictive maintenance model by training locally on sensor data from equipment across its global operations. This approach reduced downtime and maintenance costs while safeguarding proprietary data.
A Versatile Approach: Types of Federated Learning
Federated learning is not a one-size-fits-all solution. It can be adapted to suit different data scenarios, making it a flexible choice for diverse industries. Horizontal federated learning (HFL) is ideal for organizations that share the same data features across different samples, as seen in the banking example. Vertical federated learning (VFL) is suited for entities with complementary data sets, such as a hospital and an insurance company collaborating on a predictive health model. For scenarios with minimal overlap in both data features and samples, federated transfer learning (FTL) bridges the gap by combining FL with transfer learning techniques. These variations highlight FL’s versatility, enabling enterprises to deploy AI systems tailored to their specific data environments.
Driving Enterprise Success with Federated Learning
Implementing federated learning requires more than just technical expertise; it demands visionary leadership and strategic alignment. For CEOs, FL represents an opportunity to lead innovation while maintaining trust and integrity. It allows organizations to turn distributed data into actionable insights without compromising privacy or security. For CIOs, it provides a pathway to scale AI initiatives while navigating complex regulatory landscapes. CTOs, meanwhile, play a pivotal role in building the infrastructure and deploying privacy-preserving techniques like differential privacy and secure multi-party computation to safeguard model updates.
A Call to Action
Federated learning is not just the future of AI—it’s the present opportunity for enterprises to redefine what’s possible. By adopting FL, organizations can foster collaboration, drive innovation, and ensure compliance in a world where data is both a resource and a responsibility. For CEOs, CIOs, and CTOs, federated learning offers a clear path forward to balance innovation with accountability, building AI systems that inspire trust while delivering measurable value.
The competitive edge lies in the ability to act now. Enterprises that embrace federated learning today will not only lead the AI revolution but also set the standard for responsible innovation in a privacy-first world.
=================
Ashish “Monty” Mohanty is a recognized industry leader with a deep passion for leveraging AI to drive transformative innovation and solve complex business challenges. He is passionate in creating AI-driven solutions, including generative AI models, intelligent automation tools, and advanced data analytics, that deliver measurable outcomes across industries. Monty thrives on using AI to enhance customer experiences, optimize operations, and shape the future of digital transformation. His unwavering belief in the potential of AI to revolutionize industries fuels his dedication to building smarter, more connected, and forward-thinking enterprises. Monty has over 20 years of working for leading global consulting firms and is currently a Senior Vice President at Jade Global (www.jadeglobal.com), one of the fastest growing consultancies in North America.